AI in Scientific Discovery: Productivity Gains and Human Challenges
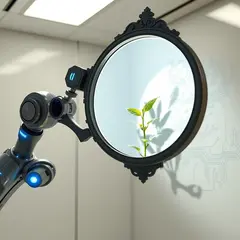
A study conducted in a materials science R&D lab reveals significant impacts of AI on scientific research and innovation. Key findings show substantial productivity gains, with AI-assisted researchers discovering 44% more materials, increasing patent filings by 39%, and boosting product innovation by 17%. However, these benefits were unevenly distributed, with top performers seeing the greatest gains. Despite increased productivity, 82% of scientists reported reduced job satisfaction due to decreased creativity and skill underutilization. The study highlights the need for balancing AI integration with maintaining scientific curiosity and job satisfaction. It also emphasizes the importance of human judgment and expertise in leveraging AI effectively, suggesting potential long-term impacts on workforce composition and scientific careers.
Introduction to AI in Scientific Research
The integration of artificial intelligence (AI) into scientific research has marked a significant turning point in the pursuit of knowledge and innovation. The thought-provoking study ¹ conducted in a materials science R&D lab provides crucial insights into the transformative potential of AI in accelerating scientific discovery. The research, led by Aidan Toner-Rodgers of MIT, offers a comprehensive look at how AI tools are reshaping the landscape of scientific innovation, particularly in the field of materials science.
The study’s findings are both exciting and challenging, revealing a complex interplay between technological advancement and human factors in the scientific process. By examining the impact of AI on various aspects of research and development, from material discovery to patent filing and product innovation, the study found a nuanced understanding of the benefits and challenges associated with AI integration in scientific endeavors.
AI’s Impact on Research Productivity and Innovation
The implementation of AI tools in the materials science lab led to remarkable improvements in research productivity and innovation. AI-assisted researchers discovered 44% more materials compared to their non-AI counterparts, demonstrating the technology’s ability to significantly accelerate the pace of scientific discovery. This increase in material discoveries translated into a 39% rise in patent filings, indicating that the AI-driven approach not only boosted initial discovery but also led to more protectable intellectual property.
Furthermore, the study revealed a 17% increase in downstream product innovation, suggesting that the benefits of AI extend beyond the initial stages of research and into practical applications. The compounds discovered with AI assistance possessed more novel chemical structures, resulting in more radical inventions. This finding is particularly significant as it counters concerns that AI might lead to incremental improvements rather than breakthrough innovations.
The overall R&D efficiency improved by 13-15%, a substantial gain that highlights the transformative potential of AI in scientific research. The study found that AI accelerated the pace of innovation and increased novelty in all three stages of R&D: material discovery, patent filing, and product prototyping. AI-generated materials demonstrated more distinct physical structures, patents introduced more novel technical terms, and there was a noticeable shift towards more radical innovation in product prototypes.
Uneven Distribution of Benefits and Performance Inequality
While the overall productivity gains were impressive, the study uncovered a significant disparity in how these benefits were distributed among researchers. The impact of AI on scientific output was not uniform across all levels of expertise. The bottom third of scientists saw minimal benefit from the introduction of AI tools, while the output of top researchers nearly doubled. This stark contrast led to a significant increase in performance inequality within the research team.
The study revealed a strong complementarity between AI and expertise, with benefits most pronounced for scientists possessing strong judgment skills. Top scientists demonstrated a superior ability to prioritize promising AI suggestions, effectively leveraging the technology to enhance their productivity. In contrast, less skilled scientists often struggled, wasting resources on testing false positives suggested by the AI.
This finding underscores the critical role of human judgment in successful AI collaboration. It suggests that rather than replacing human expertise, AI tools amplify the capabilities of highly skilled researchers. The ability to discern viable AI suggestions from less promising ones emerged as a crucial skill, highlighting the continued importance of domain knowledge and scientific intuition in an AI-augmented research environment.
Essentially, AI in scientific research acts as a powerful amplifier of human capabilities, with its success heavily dependent on the skills, domain knowledge, and judgment of the researchers utilizing it. While AI offers unprecedented potential for accelerating discovery and innovation, its effectiveness is intrinsically linked to the expertise of those who wield it, highlighting the continued importance of human insight in driving scientific progress.
Shifts in Task Allocation and Scientific Process
The introduction of AI tools led to significant changes in the allocation of tasks within the research process. The study found that AI automated approximately 57% of “idea-generation” tasks, traditionally considered the creative core of scientific work. As a result, scientists were largely reallocated to evaluating AI-produced candidates, marking a shift from open-ended exploration to a more structured knowledge production process.
This reallocation of tasks fundamentally changed the nature of scientific work, requiring researchers to develop new evaluation skills. The success of this new workflow depended heavily on researchers’ judgment and expertise. Top performers excelled in identifying viable AI suggestions, further emphasizing the complementarity between AI and domain expertise.
The shift in task allocation raises important questions about the changing role of scientists in an AI-augmented research environment. While AI tools have proven effective in generating potential research directions and hypotheses, the study highlights that human scientists remain crucial in evaluating and refining these suggestions. This new dynamic requires a reimagining of scientific training and skills development to prepare researchers for effective collaboration with AI systems.
Impact on Job Satisfaction and Scientific Careers
Despite the significant productivity gains, the study revealed a concerning trend in job satisfaction among scientists. A striking 82% of researchers reported reduced satisfaction with their work following the introduction of AI tools. The primary reasons cited for this decline were decreased creativity and skill underutilization, suggesting that the automation of idea generation tasks had a profound impact on how scientists perceive their role and value in the research process.
This reduction in job satisfaction raises important questions about the long-term attractiveness of scientific careers and the potential erosion of traditional scientific expertise. The impact on job satisfaction varied by scientist ability level, with higher productivity partially offsetting negative effects for some researchers. However, the overall trend points to a significant challenge in maintaining the intrinsic motivation that drives many scientists.
The study’s findings highlight a critical tension between productivity gains and the personal fulfillment of scientific work. There are concerns about the potential loss of wonder and delight in scientific discovery, with the risk of science becoming purely utilitarian. This shift could have far-reaching implications for the future of scientific research, potentially affecting the recruitment and retention of talented researchers in the field.
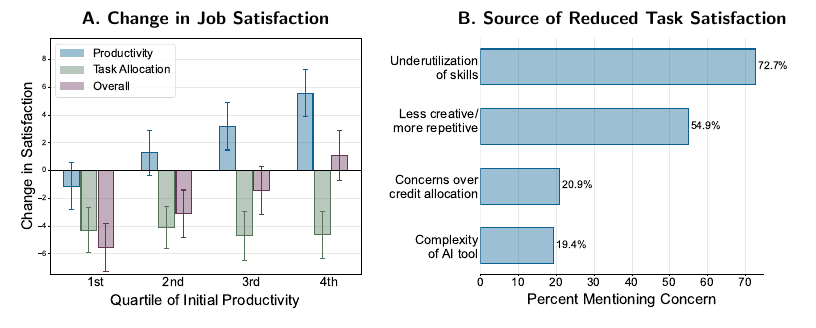
Organizational Adaptation and Broader Implications
In response to the changes brought about by AI integration, the firm adjusted its hiring practices to favor strong judgment skills. This adaptation led to the dismissal of approximately 3% of researchers, primarily those who struggled with evaluating AI suggestions effectively. Simultaneously, the overall workforce expanded through new hiring, indicating a shift in the skills and competencies valued in the AI-augmented research environment.
These organizational changes suggest that the longer-term impacts of AI integration in scientific research may be even more substantial than initially observed. The study demonstrates the importance of organizational responsiveness in maximizing the benefits of AI while mitigating potential negative effects on the workforce and research outcomes.
The findings highlight the need for a careful balance between leveraging AI for productivity gains and maintaining scientists’ job satisfaction. There is a growing recognition of the importance of preserving curiosity and wonder in scientific work, even as AI tools become more prevalent. The study underscores the need for new thinking on how to integrate AI in research while preserving the essential joy and creativity that drive scientific progress.
Future Research Directions and Potential Applications
The study opens up several avenues for future research and consideration. Long-term effects on workforce composition and equilibrium effects on the supply and demand for scientific expertise require further investigation. There is also potential for similar productivity gains in other scientific fields, such as drug discovery and genomics, where AI could play a transformative role in accelerating research and innovation.
The study raises important questions about the impact of AI on the time required to bring new materials to market and the need for adaptation in hiring and training practices. Future research should focus on developing strategies to emphasize judgment and AI-evaluation skills in scientific education and professional development.
As AI continues to evolve and integrate into scientific research, ongoing studies will be crucial to understand its long-term impacts on scientific careers, research methodologies, and the overall progress of scientific knowledge. Balancing the undeniable benefits of AI-augmented research with the preservation of scientific creativity and job satisfaction will be a key challenge for the scientific community in the coming years.
Sources:
- Artificial Intelligence, Scientific Discovery, and Product Innovation. Aidan Toner-Rodgers, MIT. November 6, 2024. https://aidantr.github.io/files/AI_innovation.pdf
[…] https://www.theaiobserver.com/ai-in-scientific-discovery-productivity-gains-and-human-challenges/ […]